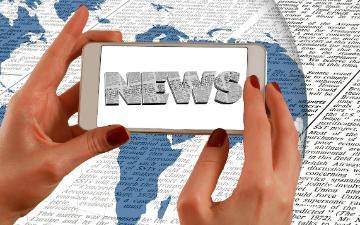
The Markkula Center for Applied Ethics received inputs from media and tech policy scholars Courtney Radsch and Robyn Caplan on the News Distribution Ethics Recommendations of late 2022. Courtney Radsch is a postdoctoral fellow at UCLA’s Institute for Technology, Law & Policy. Robyn Caplan is a visiting assistant professor of public policy and a visiting research fellow at the Sanford School of Public Policy, Duke University, and senior researcher at the Data & Society Research Institute.
In October of ’22, the Ethics Center released the News Distribution Ethics draft recommendations for public input. The recommendations were the work of the News Distribution Ethics Roundtable convened digitally at the Center by Subramaniam Vincent, director of the Journalism and Media Ethics Program. Six recommendations were made on the need for both course-grained and fine-grained disclosures by social media, search, and aggregation platforms, about how news is curated, algorithmically processed, and distributed.
February 2023 - Summary of Key Inputs Received
This section summarizes the key inputs from Caplan and Radsch.
- Develop/account for the risks of transparency: The recommendations need to have a section on risk. Risks and ethics of public disclosures need to be balanced. (Courtney Radsch)
- The risk of weaponization outweighs the ethics of public disclosure. It could be made available to accredited researchers, media development, or publisher associations, etc. (Courtney Radsch)
- News Recommender data: These disclosures should not make it harder for news organizations/journalists to compete in the platformatized media environment by letting others (PR actors, disinformation campaigns) better game the system. (Courtney Radsch)
- Recommend against blanket release of lists of entities accorded news publisher status. This yields to the idea that platforms are arbiters. (Robyn Caplan)
- Avoid binaries: The recommendations must not give the binary impression about algorithmic curation vs. human curation being good vs. bad. In most cases, all curation will be a combination of the two (stories are surfaced using algorithms, and then curated by humans). (Robyn Caplan)
- Disclose benefits of designations as “news publisher”: Around news definition disclosures (1.1), platforms could also disclose whether particular designations for news publishers gets them access to additional safety or security features. (Courtney Radsch)
- Disclose partnerships with news publishers. (Robyn Caplan)
- Geographic news source transparency: Disclosures on how platforms determine news/not-news status for entities should also help expose global inequities in status. It would be helpful for platforms to disclose which types of news sources are recommended in different markets and in different platform products. (Courtney Radsch)
- Word usage: Substitute “misused” for the word weaponized" when referring to risks of disclosure of news publisher lists. Information/culture is rarely that simplistic. (Robyn Caplan)
- Word usage: In the News Distribution Primer, choose an alternative word to “Stateful”--this is difficult to translate into other languages. (Courtney Radsch)
- Disclose partnerships with fact-checking entities: There should be a recommendation about fact-checkers, how they are used on platforms, which organizations are partners with a geographic and linguistic breakdown, how fact-checks influence moderation/distribution, and what (if anything) is done to prevent fact-checked articles from spreading further. (Courtney Radsch)
- New section needed for disclosures on monetization: "Fake news farms" are engaged in the business because it is profitable. Global majority (formerly termed "Global South") publishers lament the inability to monetize their content on platforms which reduces incentives to use or improve them. (Courtney Radsch)
- Narrow scope to reduce the volume of disclosures: These recommendations, if implemented, may require a lot of data to come out which consume time and resources for smaller entities. For example, disclosures about actions taken on the news articles violating policies: Reduce this to release for specific time periods only, for example, and not ongoing. (Robyn Caplan)
Detailed inputs and related context below, by section.
Approach
Our text
We rely on three paradigms to motivate and clarify our recommendations: rights, harms, and obligations regarding democratic discourse. Human rights are often used to motivate ethical approaches around original principles that have broad global consensus. Harm-based standards, legal or regulatory requirements, policies, and design are widely adopted, for instance, in online content moderation. As noted earlier, the content, quality, and tone of discourse is greatly determined by the news (and its ethics) that publishers produce and its distribution by aggregators and platforms; what ethical obligations exist around discourse? These three paradigms are interconnected and can be in tension with one another, for example, defining the line between someone’s right to expression and harm to another person.
Inputs
Courtney Radsch: In addition to the Harms, there should be consideration of Risk. Risk would focus on likelihood and thus the enabling environment.
NDE Primer
Our current draft text
We distinguish the term “Surfaces.” Surfaces may be either unpersonalized, stateful, or personalized.
Stateful: The product is showing you something based on where you left off with a story, or what you last searched, or where you currently are. There is no inference in your implicit or explicit interests, just recalling from a log what you've done and reflecting it back to you.
Inputs
Courtney Radsch: “Stateful” is a less-than-ideal term since, at first glance, it seems to refer to state intervention or influence, and it would be difficult to translate this term into other languages. Move to an alternative term.
1.1 Definitions Disclosures
We recommended
-
-
How does your platform define “news” for both curation and algorithmic classification purposes?
-
How does your platform currently accord “News” site or “News publisher” status to any entity?
-
How does that designation affect access for (or promotion of) news publishers
- Access: Access to platform policy, exemptions, resources, business partnerships, etc. For e.g. some platforms are offering news publishers exemptions of particular kinds for boosting/advertising of content (ad policy) otherwise deemed as "political." Another access example is just registering formally as a publisher to be included in listings like News Tab or Showcase. Some news publishers get beta access to features/other special access to distribution measures.
-
Does your platform define “who is a journalist” for curation and algorithms and if so, what is the definition or standard?
-
Definitions for the following as used by the platform. “Curation,” “Location,” “Topics,” “Trends/Trending,” and “News categories” used on feeds.
-
How does your platform define and/or detect “Opinion” from news publishers?
-
Inputs
Courtney Radsch: This is useful and seems doable, as it is focused on policy. It would also be helpful to know whether such designations provide access to additional safety or security features, since online harassment, coordinated reporting campaigns, and weaponized DMCA and GDPR complaints often target news outlets and journalists on these platforms, and are often specifically designed to influence content moderation and distribution systems.
See for example, AI and Disinformation: State-Aligned Information Operations and the Distortion of the Public Sphere, https://papers.ssrn.com/sol3/papers.cfm?abstract_id=4192038
Robyn Caplan: This is a good starting point for disclosure recommendations.
- Asking platforms to disclose more information about *how* they are making these determinations (human or algorithm) may also help for understanding any global inequities that may exist in the determination of news/not-news (i.e. where are platforms directing their resources towards understanding the news landscape).
- It would also be useful that platforms disclose any sort of existing partnership they may have with a publisher (through, for instance, Twitter Blue, etc).
1.2 Curation Disclosures
We recommended
Note: By ‘curation’ we specifically refer to human beings on the platform side, employed or contracted, choosing and/or ordering articles as well as news sources, for particular surfaces.
-
-
- Disclose surfaces and ways where human curation is a part of the platform
- Disclose surfaces and ways where human training models are used for curation
-
Disclose principles and policies that guide curation
-
Disclose list of curated topics in each <period/reporting cycle>
- Example of “Topic”: Racial Justice, Housing, Food, etc. When you click those links it shows all stories in that topic. Sometimes a term represents a developing story itself like "Infant formula" but those go away after a while. Some terms are standard and product people are posting them on top. For example, “COVID-19” is top on many news product surfaces.
-
Which news stories did human curators give prominence to (display at the top of page/feed) per topic/moment/event/#trend each <period/cycle> without algorithmic assistance?
-
Which news stories did human curators give prominence to (as in a. above) but with algorithmic assistance?
-
Inputs
Robyn Caplan: I agree with the spirit of these disclosures, but I do think it lends itself to giving a binary of good/bad of algorithmic curation vs. human. In most cases, all curation will be a combination of the two (stories are surfaced using algorithms, and then curated by humans).
Being clear about that is helpful, but I don't want us to set ourselves up for positioning one system as good and the other bad. They both have their benefits and drawbacks.
Items 3-4 seem unrealistically detailed, and few platforms except the best resourced would realistically even be able to comply. Furthermore, we don't require that news media sources provide lists of topics they give prominence to on, for example, their front pages.
Courtney Radsch: We need a geographic news source transparency requirement. It would be helpful for platforms to disclose which types of news sources are recommended in different markets and in different products, such as Facebook's program Free Basics or in Pakistan versus Lebanon.
For example, in my interviews with journalists in LDCs they are frustrated by two things: 1) they do not show up as leading sources in searches for news occurring in their countries or global sources like BBC, CNN, NYT, show up first; and 2) they do not show up in results for news about their countries to users outside of their countries (e.g. Ukrainian or Syrian sources showing up alongside global reporting on the wars).
1.3-News Publishers
We recommended
Note: We recognize that any release of lists could be weaponized. The generation of such lists needs to be implemented with guardrails to mitigate disclosure risks. Each platform may have its own approach to this and we recommend they document it as part of this disclosure. For instance, some platforms may document their appeals process for decisions and disclose that along with these lists.
By market (US, Europe/countries, Asia/countries, US-local markets, etc.
-
-
List of entities accorded news publisher status on curated products or by human curators
-
List of entities whose content was recommended algorithmically as “News”
-
List of publishers whose stories (claims in stories) were disputed and failed third-party fact-checkers (false or pants on fire)
-
List of publishers who violated content policies and details
-
List of publishers who were demonetized and grounds
-
List of publishers whose accounts were suspended or deplatformed
-
List of publishers whose accounts were restored after suspension
-
List of publishers whose content triggered top #trends data (per cycle)
-
List of publishers who are labeling News vs. Opinion
-
List of entities whose content is not curated or not recommended as “News” but who have asked for News Publisher status (long tail), i.e. entities who have asked for, but never heard back and were not denied
-
List of entities who requested news publisher status by formal means but not granted with grounds (junk publishers, deception, imposters, etc..), i.e. news entities that have been explicitly denied status
-
Inputs
Courtney Radsch: The risk of weaponization outweighs the ethics of public disclosure. It would be great if platforms would track and collect this data (though I think that is very unrealistic except again for the biggest and wealthiest ones). Instead of public disclosure, it could be made available to accredited researchers, media development, or publisher associations, etc.
Robyn Caplan: Substitute “misused” for weaponized." “Weaponized” lends itself towards an information warfare approach in which some entities (right now, the U.S.) are conceived as “good actors” versus “bad actors.” Information/culture is rarely that simplistic. Some of this is going to be difficult to actually do, and will end up pulling in much larger amounts of publishers than you think.
For instance, almost every publisher/creator has been demonetized at some point by platforms like YouTube. YouTube frames its demonization guidelines very conservatively and it's largely done through algorithms. Unless there is an active partnership with YouTube and/or the site is “whitelisted,” you'll get demonetized if you cover anything even remotely political. I'd recommend against doing this because then you're fully positioning the platform as arbiter (and discrediting virtually any publisher that has a presence on these sites).
1.4-News Recommenders
We recommended
By curated topics/trends/moments/events (e.g. for Twitter) and/or
By market (U.S., Europe/countries, Asia/countries, U.S.-local markets)
- List the top #n most prominent recommendations
- List the top #n impressions
- List the top #n most viewed
- (a,b,c) List of top #n most prominent recommendations/impressions/views only from news publishers (entities that have identified themselves as news publishers, or that the platform otherwise considers as a news publisher)
- (a,b,c) List of top #n most prominent recommendations/impressions/views only from organic human accounts (exclude accounts of journalists connected with one of the news publisher entities)
- List the top #n most shared posts (retweets/shares)
- List of top #n most shared posts only from news publishers
- List of top #n most shared posts only from organic human accounts (exclude accounts of journalists connected with one of the news publisher entities)
- Does the platform maintain a quantitative measure of the percentage of content that got into distribution from the curation side vs. the algorithmic recommendation side? If so, define the measure and disclose numbers for recent measurement periods.
(Note: We recognize that there are going to be standardization challenges for terminology such as topics, trends, moments, events, etc., that will make normalized comparisons difficult across platforms. One of the goals of this effort would be to move to standardization after some cycles of disclosure and learning have taken place.)
Inputs
Courtney Radsch: What are the risks that the provision of this information would make it even harder for news orgs/journalists to compete in the platformatized media environment given that moderation mercenaries from PR firms to government actors will undoubtedly mine this list to figure out how to better game the system? Furthermore, these systems are so susceptible to manipulation that this could risk distorting the point of the whole effort. Also, how does this actually link to the obligation for fostering discourse? Discussion about marginalized topics might not get trending.
1.5-Remedial Actions
We recommended
Below, per day/week/per market:
- List of news posts/articles referred to fact-checkers and flagged as disputed and their retweet/share #n counts after the flagging happened.
- List of news posts/articles that violated content policies and were acted on in any way–engagement limited, labeled, demonetized, deplatformed, etc.
(*Note: Items 3 and 4 below tagged “News Publisher Corrections”, are related to corrections in news articles at the publishers’ end. Currently, news aggregators and platforms do not have standardized visibility into data showing corrections in real time. We acknowledge these two items are futuristic.)
- *News Publisher Corrections: List of most prominent recommendations of news items that were later corrected by publishers, and the relative distribution data for those posts (This is to capture post-correction distribution (tweets, retweets) for the same story. Let's say WaPo posted the first story at 9:01a.m. 5/16. And it got {x-impressions,y-shares/retweets,z-something} distribution metrics. At 12:02p.m. they made a correction and posted that. Disclose the distribution for the correction in the same {x,y,z} terms)
- *News Publisher Corrections: List of most shared posts of news items that were later corrected by publishers, and the relative distribution data for those posts (same as above, except engagement metric changes from impressions to sharing)
Inputs
Robyn Caplan: Though I would find this data very useful, it's going to end up becoming a pretty large net.
Courtney Radsch: This sounds like an interesting study but publishers themselves don't even have a way to curate all of their stories by correction, for example. This set of recommendations would be great in a perfect world, but it makes the broader effort look too unrealistic. News organizations need to get their own house in order before making the platforms responsible for doing what they themselves do not. #2 would be very useful for publishers but seems to be more likely to be possible in a defined time period or for an issue, rather than ongoing reporting.
Courtney Radsch: There should be a recommendation about fact-checkers, how they are used on platforms, which organizations are partners with a geographic and linguistic breakdown, how fact-checks influence moderation/distribution, and what (if anything) is done to prevent fact-checked articles from spreading further.
1.6 Opinion Policy
We recommended
Policy
- Are you automatically detecting opinion as distinct from reporting? If so, is your system itself designating an opinion label?
- Is your platform separating opinion feeds for topics/developing stories/etc.?
- Is your platform passing opinion label metadata (such as schema.org labels, when present) downstream to the news feed/discovery surfaces for users to see as a label?
- How is your platform handling conflict between a publisher-supplied label and your own auto-detection? (E.g. Publisher says “reportage or news,” but your detection says “opinion”)
- Is your platform subjecting opinion journalism to any form of vetting? If so, what? (e.g. internal validation/assessment for factual basis, or fact-checking.)
Data
By curated topics/trends/moments/events AND/OR
By market (U.S., Europe/countries, Asia/countries, U.S.-local markets)
-
-
List of top#n most prominent recommendations/impressions/views of opinion posts from news publishers
-
List of top#n most shared opinion posts from news publishers
-
List of news publishers labeling opinion content formally whose stories you are distributing
-
Inputs
Courtney Radsch: The policy disclosures seem reasonable. Data disclosures do as well but #3 should be first.
Other Inputs
Courtney Radsch: This is a great effort but parts of it seem unrealistic or overly focused on a couple of big platforms. I would suggest adding a section on monetization since a lot of "fake news farms" are engaged in the biz because it is profitable, and a lot of "Global South" publishers lament the inability to monetize their content on platforms which reduces incentives to use or improve them.
View or download the News Distribution Ethics Recommendations.