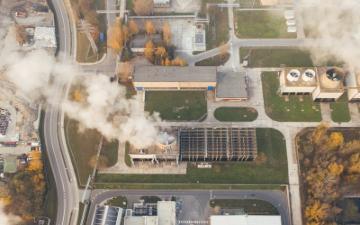
Leila Scola was a 2019-20 Environmental Ethics Fellow at the Markkula Center for Applied Ethics.
Because AI is now being used for nearly all major computing tasks in the modern world, examining the relation between energy consumption and computational output of AI is extremely relevant. Despite the large energy draw AI takes to train and run, the beneficial calculations of where to save energy and how to consume energy, in addition to other calculations being made, outweigh the negatives of training and running AI.
Machine learning algorithms make use of GPUs cores instead of the CPUs. All computers contain a CPU, or central processing unit. CPUs are a part of circuit boards and interact with other pieces of hardware, calling information from a hard drive to respond to a user’s commands to execute a series of successive interactive commands. All computers also have GPUs, or graphics processing units, which are also used to drive most AI. GPUs break complex problems into thousands or millions of separate tasks that it works on in parallel, which is why they are used to train AI and process related data. It is good at taking many sample points and finding correlations between them. CPUs have several cores, low delay, and can serially process, while GPUs have many cores, feed information quickly and parallel process.[1] Since a GPU utilizes the entire chip at the same time, pipelining and executing its compute tasks asynchronously, a GPU can complete more work per Joule of energy, while a CPU can finish tasks faster.[2]
Like any piece of technology, GPUs are powered by electricity, calculated in kiloWatts per hour. This energy typically requires the burning of various fossil fuels, primarily natural gas and coal, resulting in CO2 emissions.[3] The biggest energy draw of AI is its training. This requires anywhere from days to months of feeding data into a GPU (or many GPUs) to run calculations and slowly refine identification markers, which are specific data points that the GPU uses to compare to incoming data. For example, if one is attempting to locate birds in photos, they will feed thousands of photos of birds into a GPU, which breaks each photo into pixels, then differentiates color patterns to locate the coloring of a bird against its environment. To ensure the AI accurately identifies birds, one will feed the GPU images of birds in front of trees, or in dead grass, where they might blend in, rather than against a blue sky, making the model refine its identification techniques.
Studies have determined that it takes a fair amount of energy to train an AI model.[4] One example indicates that training a model to be able to understand and process human language produces 626,155 lbs of CO2 emissions over the course of 3.5 days. This is an environmental impact equivalent to the lifetime of five cars.[5] Note that, on average, one kWh produces a little over one pound of CO2 due to the burning of natural gas and coal.[6] This number was found by adding the power drawn from all the sockets on the machine and multiplying it by the Power Usage Effectiveness index. While the power consumption here may seem excessive, one must consider how much power is drawn from an average laptop or computer that runs primarily on CPU computation. This training, being one of the worst ones, uses .0055 W/hr. The average laptop uses 50-100 W/hr and the average desktop uses 200 W/hr.[7] On average, AI models use power comparable to household devices, yet can run calculations that will save energy, power, time, and money.
Since about 2017, major tech companies have promised to reduce their carbon footprint and have used AI to make drastic changes to the way they operate. The top AI companies include Amazon, Apple, Facebook, Google, Microsoft, and others.[8] Apple met its goal for 100% renewable energy operations for their facilities in 2018, relying on utility-scale solar projects, and committing twenty-three additional manufacturing partners to this goal.[9] Apple alone avoids 2.1 million metric tons of CO2 emissions from entering the atmosphere, reducing greenhouse emissions by 54% from its facilities worldwide.[10] Facebook has stated it is committed to 100% renewable energy operation by 2020 and has purchased 2 GW of renewable energy.[9] Microsoft is one of the most energy efficient companies in America with 83% of its power coming from hydroelectric power, while using biogas, biomass, and hydropower and solar power to keep their lights on.[11] Google met its goal of 100% renewable energy for global operations in 2017 and 2018 through Purchase Power Agreements for solar and wind projects.[12] Altogether, Amazon, Facebook, Microsoft, T-Mobile, AT&T, Google and Apple account for more than half of renewable power purchases that occur worldwide during a given year.[13] Amazon will build three new wind farms by 2021 that will generate 500,997 megawatt-hours of electricity per year (enough for a small city).[14] These companies then pump the extra power back into the municipal power grid, countering energy they use and generating excess to be distributed across communities. This means that most of these companies are not only leaders in AI, but leaders in green energy consumption.[15]
Data centers account for 2-3% of electricity demand in developed nations.[13] From 2000-2005, energy consumption (in the United States) grew 90%, then 24% in the next 5 years, then another 4% in five more years and was predicted to have grown about 4% in the last 5 years.[16] Despite this, data centers have increased computing power many times over, thus demonstrating their vastly increased efficiency. This is thanks to measures taken at companies such as Google. Google has used AI to take snapshots of its data center cooling system from thousands of sensors every five minutes and feed the information into a neural network.[17] Tweaks to reduce energy consumption are identified and checked by the local control system, then implemented. This has resulted in a 40% reduction in the amount of energy used for cooling, slowly improving from 15% upon implementation.[18] In addition, other companies have been using AI to identify where energy is unused to export it back to the grid, transferring back 80% of energy along power lines, reducing CO2 by 30% in one trial. This can be used to help buildings store energy during off-peak hours that can be discharged later to lower the burden on the grid. Currently, there are hybrid energy storage systems that generate energy from heat and store it in various systems (ranging from solar panels to batteries) when it is needed, reducing energy consumption by 14-40%. This allows buildings to store energy during off-peak hours and discharge it during peak hours, lessening the burden on the grid. In power plants, energy can be stored instead of lost in cooling towers. This type of energy conservation requires analysis and prediction only AI can manage.
By allowing AI to make decisions of where to conserve energy on the grid and predict where to turn the power off and where to transfer it to, it is possible to produce up to 80% of energy used in a certain system from its own hybrid system. Smart plugs, batteries and AI managing surges in energy demand account for this. For example, AI could make the decision to temporarily switch off all the fridges in an area rather than call on generation from fossil fuel plants. In short, not only can effective use of AI systems within data centers result in fairer energy distribution, but as a model trains, it can drastically reduce overall CO2 emissions, not only countering its own energy consumption, but that of the system it analyzes. As a whole, this system could reduce carbon emissions up to 30% from municipal energy consumption. Americans alone use about 11,000 kWh per capita per year, equivalent to about 11,000 lbs of CO2.[19] If we could reduce this number up to 30%, we could slow the rise of global warming that is endangering our planet’s health. Unlike computers and other technology we use for our own enjoyment and entertainment, AI can pave the way to a more sustainable and equitable future, and reduce CO2 emissions by 4% worldwide.[20]
Use of smart technology will be able to help humans allocate energy more efficiently. AI will help us choose where to send our power, choose how to store excess power, and choose how to run systems in order to conserve energy as much as possible. The future of technology indicates that AI will be able to make these decisions to reduce our energy consumption while the number of devices used increases. If we are willing to run laptops and computers that consume energy comparable to the energy consumed by AI, we should be willing to run the AI that will help reduce energy while running. As our demand for energy increases, and the world of AI use expands, we should utilize these machines that have been helping us to manage energy efficiently. They are able to find patterns and make finite decisions based on these patterns of where to save energy in unique situations, ones where humans either cannot see the details or cannot react fast enough. With the help of large tech companies, we are heading in the direction of hitting carbon neutral and creating devices that can help us make improvements in terms of energy use, as well as other portions of our lives. These decisions will ultimately result in the reduction of carbon emissions we hope to see.
- Caulfield, B. (2019, October 18). Difference Between a CPU and a GPU? Retrieved from https://blogs.nvidia.com/blog/2009/12/16/whats-the-difference-between-a-cpu-and-a-gpu/
- Buyukisik, H. T. (2018, October 18). Why are GPUs Claimed to be More Power/Energy Efficient Than CPUs. Retrieved March 5, 2020, from https://www.quora.com/Why-are-GPUs-claimed-to-be-more-power-energy-efficient-than-CPUs
- Fossil fuel. (n.d.). Retrieved from https://www.sciencedaily.com/terms/fossil_fuel.htm
- Strubell, E., Ganesh, A., & Mccallum, A. (2019). Energy and Policy Considerations for Deep Learning in NLP. Proceedings of the 57th Annual Meeting of the Association for Computational Linguistics. doi: 10.18653/v1/p19-1355
- Peckham, O. (2019, July 13). AI's Carbon Footprint May Be Bigger Than You Think. Retrieved December 2019, from https://www.enterpriseai.news/2019/07/11/ais-carbon-footprint-may-be-bigger-than-you-think/
- Clayton, J. (n.d.). 1 kilowatt-hour. Retrieved from https://blueskymodel.org/kilowatt-hour
- How much power does a computer use? And how much CO2 does that represent? (n.d.). Retrieved from https://www.energuide.be/en/questions-answers/how-much-power-does-a-computer-use-and-how-much-co2-does-that-represent/54/
- Botha, M. (2019, January 28). The 15 most important AI companies in the world. Retrieved from https://towardsdatascience.com/the-15-most-important-ai-companies-in-the-world-79567c594a11
- How the tech giants are fueling a solar revolution. (2019, August 6). Retrieved from https://www.renewableenergyworld.com/2019/08/06/how-the-tech-giants-are-fueling-a-solar-revolution/#gref
- Apple now globally powered by 100 percent renewable energy. (2020, February 26). Retrieved from https://www.apple.com/newsroom/2018/04/apple-now-globally-powered-by-100-percent-renewable-energy/
- 100 of the Most Energy Efficient Companies in America. (n.d.). Retrieved from https://www.electricchoice.com/blog/100-energy-efficient-companies/
- Statt, N. (2019, February 26). Google and DeepMind are using AI to predict the energy output of wind farms. Retrieved December 2019, from https://www.theverge.com/2019/2/26/18241632/google-deepmind-wind-farm-ai-machine-learning-green-energy-efficiency
- Walton, R. (2018, November 15). Big tech companies are becoming the top buyers of green energy to meet data needs: BNEF. Retrieved from https://www.utilitydive.com/news/big-tech-companies-are-becoming-the-top-buyers-of-green-energy-to-meet-data/542256/
- Vetter, D. (2019, October 28). Big Techs Renewable Energy Spend: Is This Greenspinning? Retrieved from https://www.forbes.com/sites/davidrvetter/2019/10/27/big-techs-renewable-energy-spend-is-this-greenspinning/#383b70b6259f
- Hanley, S. Big Tech Companies Are Driving Demand for Renewable Energy. (2018, November 16). Big Tech Companies Are Driving Demand For Renewable Energy. Retrieved from https://cleantechnica.com/2018/11/17/big-tech-companies-are-driving-demand-for-renewable-energy/
- Hölzle, U. (2016, June 28). Data centers get fit on efficiency. Retrieved from https://blog.google/outreach-initiatives/environment/data-centers-get-fit-on-efficiency/
- Ranger, S. (2019, January 19). Google just put an AI in charge of keeping its data centers cool. Retrieved from https://www.zdnet.com/article/google-just-put-an-ai-in-charge-of-keeping-its-data-centers-cool/
- Evans, R., & Gao, J. (2016, July 20). DeepMind AI Reduces Google Data Centre Cooling Bill by 40%. Retrieved December 2019, from https://deepmind.com/blog/article/deepmind-ai-reduces-google-data-centre-cooling-bill-40
- “U.S. Energy Information Administration - EIA - Independent Statistics and Analysis.” How Much Electricity Does an American Home Use? - FAQ - U.S. Energy Information Administration (EIA), www.eia.gov/tools/faqs/faq.php?id=97&t=3.
- Mehta, A. (2019, June 24). Can AI light the way to smarter energy use? Retrieved December 2019, from http://www.ethicalcorp.com/can-ai-light-way-smarter-energy-use