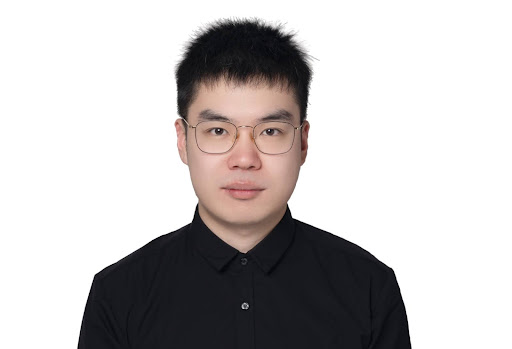
Responsible AI: Ensuring Fairness in the Age of Automation
Imagine an AI system that helps decide who gets access to vital information, receives critical assistance, gets hired, and more. These systems have the potential to shape our lives dramatically. But what happens if their decisions are biased? Can we trust AI to make decisions that affect our future if not designed responsibly? This is the critical issue at the heart of responsible AI.
Artificial Intelligence (AI) is revolutionizing sectors such as healthcare, business, and education by enabling machines to perform tasks traditionally requiring human intelligence, like solving problems and making decisions. But with this immense potential comes significant responsibility. Like any human-driven technology, AI systems can inadvertently perpetuate societal inequalities if not carefully designed. How can we ensure that AI advances innovation and does so in a way that is fair, ethical, and aligned with human values?
Computer Science and Engineering Ph.D. student Xuyang Wu’s research on responsible AI addresses this important question. His work focuses on identifying and mitigating the biases that often emerge in AI systems—biases that can have real-world consequences. By examining these biases and developing frameworks for responsible AI design, Xuyang is helping ensure that AI technologies prioritize ethical, legal, and societal considerations. This approach is not just about making smarter machines; it's about making sure those machines make decisions that promote fairness, equity, and positive outcomes for all people.
What Is Responsible AI?
Xuyang Wu defines responsible AI as “an AI system that prioritizes ethical, legal, and societal considerations, ensuring that these technologies align with human values and promote positive outcomes for individuals and society.”
The term may sound technical, but its implications are deeply personal. Think about the everyday decisions AI systems make today, and how many more critical decisions they will make at the peak of automation. If AI systems aren't designed with care, they can reinforce inequalities and foster unfair practices. This is where Xuyang’s work comes in.
His research focuses on tackling fairness challenges in AI across various fields. For instance, he studied the fairness of Large Language Models (LLMs) such as GPT and Llama 2 in text-ranking tasks [1]. He found that these models often show biases related to gender and geography, giving unfair advantages to certain groups over others. This type of bias in AI can have far-reaching consequences—affecting everything from hiring processes to access to information. However, Xuyang’s work is developing empirical benchmarks for evaluating these models to ensure they are fair and equitable.
The Hidden Biases in AI: What’s at Stake?
When we think of bias, we might picture a person intentionally acting unfairly, but bias can often be subtle and unintentional in AI. AI systems are only as good as the data they are trained on. If that data is biased—whether due to historical inequalities, skewed representation, or systemic problems—then the AI will likely reproduce these biases. Imagine a hiring algorithm that learns from a dataset where most successful candidates are men. It might unintentionally favor male applicants, even if the hiring managers did not intend to discriminate.
Data Bias: AI systems are only as good as the data they are trained on. If the training data is not representative of the real-world population or is skewed, the system will inevitably reflect those biases. Causes include historical inequalities, overrepresentation or underrepresentation of certain groups, and data collected from narrow or biased sources.
Algorithm Bias: Bias introduced by the algorithms or models themselves, regardless of the quality of the data. Causes include optimization goals that prioritize accuracy over fairness, assumptions or simplifications made during model development, and imbalanced model parameters or features that favor certain groups [2].
Both types of bias demonstrate the critical need for careful data selection and thoughtful algorithm design to avoid reinforcing social inequalities. AI has a significant impact—it can influence decisions ranging from hiring practices to healthcare access. Therefore, it's crucial to ensure these systems are equitable and just.
The Road Ahead: Why We Need to Prioritize Responsible AI
The significance of responsible AI research is clear. Ethical and unbiased AI development is essential to prevent unintended consequences, promote fairness, and build public trust in these technologies. As AI becomes an increasingly integral part of our daily lives, its impact will only grow. Ensuring that AI systems are aligned with human values, transparent, and beneficial to society is crucial.
Xuyang Wu’s work exemplifies the importance of research in this field [1, 2, 3]. But his efforts are not alone. The new interdisciplinary program in Responsible Artificial Intelligence (R-AI), led by Dr. Yi Fang at the School of Engineering, is a step in the right direction. The program aims to ensure AI advancements are safe, equitable, transparent, and beneficial for all by fostering interdisciplinary research, promoting ethical AI education, and cultivating partnerships.
The call to action is clear as Xuyang and others continue to push for progress. We must all be part of the conversation. Whether you’re a student, a researcher, or simply consuming technology, actively contributing to the future of AI is essential.
The Challenges in Creating Fair AI
How Responsible AI Is Transforming Real-World Applications
|
---|
References:
[1] Wang, Y., Wu, X., Wu, H. T., Tao, Z., & Fang, Y. (2024, June). Do Large Language Models Rank Fairly? An Empirical Study on the Fairness of LLMs as Rankers. In Proceedings of the 2024 Conference of the North American Chapter of the Association for Computational Linguistics: Human Language Technologies (Volume 1: Long Papers) (pp. 5712-5724).
[2] Wu, X., Li, S., Wu, H. T., Tao, Z., & Fang, Y. (2025, January). Does RAG Introduce Unfairness in LLMs? Evaluating Fairness in Retrieval-Augmented Generation Systems. In Proceedings of the 31st International Conference on Computational Linguistics (pp. 10021-10036).
[3] Wu, X., Wang, Y., Wu, H. T., Tao, Z., & Fang, Y. (2024). Evaluating Fairness in Large Vision-Language Models Across Diverse Demographic Attributes and Prompts. arXiv e-prints, arXiv-2406.