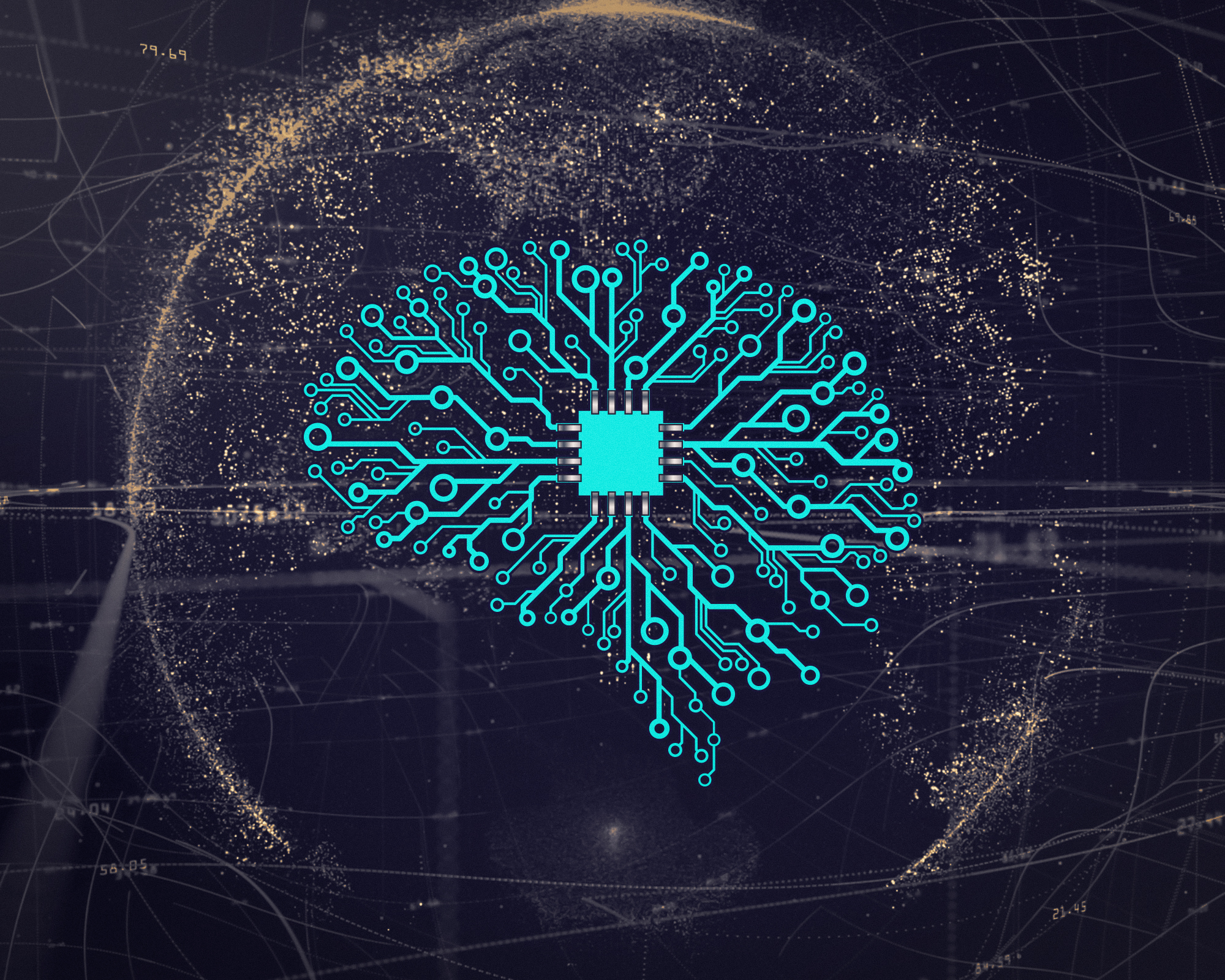
Unhealthy AI
Image by Mike Mackenzie via Flickr
Imagine, pre-pandemic, making an appointment for a routine physical. The only available time slot is 11 a.m. When the day comes, you skip the appointment because you can’t get the time off from your hourly job to travel there and back; pay for those couple of hours meant covering rent that month.
The office administrator notes your no-show in your file. So now, when you call to re-book, the clinic looks at your record of unreliability and double-books the appointment window, assuming you’ll skip it again. When you do make it in, it takes the doctor an extra hour to see you. And your unpaid two hours off has suddenly become three.
This scenario might sound familiar to many African Americans, who are more likely to live farther from their place of employment, face job discrimination when looking for better-paid work, and work in less stable occupations. According to this August 2019 Forbes article, even in the midst of the healthiest job market in years last summer, blacks in their prime earnings years were consistently less employed than whites—about 75% versus 80%.
As this relates to healthcare access, people who failed to show up in the past are scheduled in undesirable and overbooked appointment slots.
It’s unsurprising, then, that artificial intelligence, or AI, seemed like the perfect solution to create a non-biased scheduling system. But, as it turns out, AI was just a reincarnation of our problematic social constructions in computer form.
In new research, Michele Samorani, SCU management and information systems assistant professor, found that healthcare scheduling algorithms were actually perpetuating the same tendencies of their human predecessors. “Black patients are less likely to show up because race is correlated with socio-economic factors such as employment status and lack of transportation, which are linked to the no-show risk,” Samorani says. “Just like those who have a low credit score are less likely to be approved for a loan, those who have failed to show up in the past are more likely to be assigned an undesirable appointment slot in the future.”
What we ask AI to do says a lot about us—like deciding who should wait longer to see a doctor. That’s because computers are machines that are programmed to learn and develop artificial intelligence from our past successes and failures, says co-author Michael Santoro, Leavey School of Business professor and business ethicist. “In the process of learning and becoming intelligent they can perpetuate or, as in our study, exacerbate discrimination,” he says. “So if a tech company makes it hard for women to succeed in the workplace and it designs a hiring algorithm to help it sort out the potentially best employees, the hiring algorithm will make it less likely to hire women thereby exacerbating the original discrimination.”
So the team—also comprised of Haibing Lu, Information Systems & Analytics Department chair; Linda Goler Blount, president and CEO of The Black Women’s Health Imperative; and Virginia Commonwealth University Assistant Professor Shannon Harris—sought a solution. The original algorithms used in appointment scheduling were meant to do two things: minimize waiting time for patients and maximize the time a doctor spends with patients Instead of erasing race from the equation, they brought it front and center.
Samorani explains, “We changed the objective used to schedule appointments: Instead of minimizing the expected waiting times of all patients, let’s minimize the waiting time of the racial group who’s expected to wait the longest. This way, any disparity in waiting times is penalized.” As a result, the team found an algorithm that did not decrease efficiency in appointment scheduling but skyrocketed it in terms of fairness.
Healthcare facilities began reaching out to replace their old algorithm with this new one. It meant that instead of black patients being overbooked and waiting longer, white patients would have to share in the wait in order to create a more equitable system. Black patients would be able to receive the more immediate medical attention their white counterparts have been receiving for centuries.
Samorani is now working on additional research to assess whether, in addition to waiting longer at the clinic, black patients also wait longer to get an appointment in the first place. “Our research is only possible thanks to a diverse, multidisciplinary team composed of experts in data science, operations management, and business ethics, as well as a public health advocate. I believe that this work truly embodies the Santa Clara spirit – using technology to fix the injustices that penalize the most vulnerable among us.”