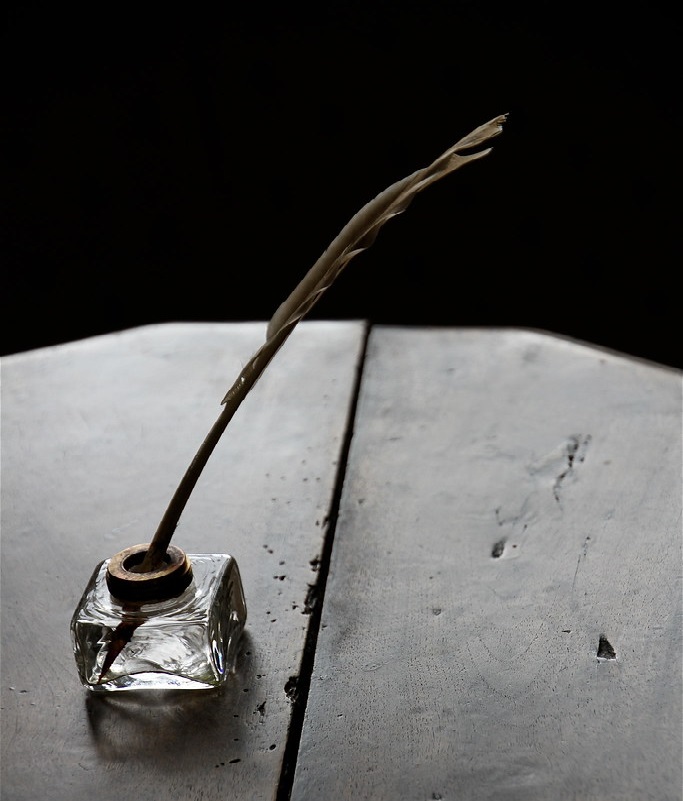
On Avoiding Pitfalls
Irina Raicu is the director of the Internet Ethics program (@IEthics) at the Markkula Center for Applied Ethics. Views are her own.
It is a truth universally acknowledged—or at least a belief shared by many artificial intelligence and machine learning researchers—that, given a vast database and sophisticated modeling, an algorithm will be able to predict the behavior of individual human beings.
Jane Austen might seem like the wrong authority to turn to in order to dispute this. But she does have some relevant insights on the topic.
You might remember that in her novel Pride and Prejudice Austen features a heroine named Elizabeth who has a lot of interactions with a character named Mr. Darcy, whom she eventually marries. Initially, though, over the course of several meetings, and from various stories that other characters tell her, Elizabeth collects information about Darcy that leads her to dislike him—and to believe that he dislikes her in return. As a result, she is stunned when he eventually, suddenly, proposes to her. That was not the behavior that she would have predicted, from him.
Of course, her prediction was based on a limited amount of information about him—some of it collected and delivered to her by motivated (biased) sources. The novel is thus a cautionary tale relevant to the unwarranted pride and prejudice embedded in the belief that AI can effectively anticipate individual people’s behavior.
In the Fall of 2020, two Princeton professors co-taught a computer science course titled “Limits to Prediction.” As they explained in a very useful pre-reading essay, “researchers and companies have made many optimistic claims about the ability to predict phenomena ranging from crimes to earthquakes using data-driven, statistical methods. These claims are widely believed by the public and policy makers.” Looking at the accuracy of current predictive algorithms, however, the professors (Arvind Narayanan and Matt Salganik) ask whether there are “practical limits to predictions that will remain with us for the foreseeable future,” and explain the importance of determining this: “If we are entering a world where the future is predictable, we need to start preparing for the consequences, both good and bad. If, on the other hand, commercial claims are overhyped, we need the knowledge to push back effectively.”
In their essay, the professors offer several hypotheses about the limits of prediction. In regard to predictions about human beings’ actions, one of them seems particularly applicable: they call it “Shocks.” “Life trajectories,” they write, are “sometimes upended by the kinds of inputs that seem likely to remain unmeasurable for the foreseeable future: a lottery jackpot; an accident; a crime of passion committed in the heat of the moment; a college admission for which one just made the cut. What is unclear is how common these are in the typical life course and to what extent they limit predictability.” The range of “shocks” that substantially alter human behavior but are likely to remain unmeasurable inputs is much broader, though, and some are much more common: the birth of a child; emigration; illness; heartbreak; travel to different countries, etc.
In Pride and Prejudice, for example, we find out that Darcy’s behavior is greatly changed in part by the shock of Elizabeth’s stunned and angry refusal of his first marriage proposal. His second goes much better.
Of course, Elizabeth had not correctly anticipated the first one; that might be due in part to another factor that the professors list as a separate hypothesis for limits to predictions: “Unobserved or unobservable inputs.” In regard to human beings, this seems more of an axiom. As Narayanan and Salganik note, “relevant attributes are often unavailable for prediction”; as an example, they add that “as long as people’s thoughts remain inaccessible to predictive algorithms, that will impose limits to the predictability of some types of events.” In Pride and Prejudice, Mr. Darcy is often accused of being inscrutable—and in Jane Austen’s world many kinds of “inputs” were not to be said or done. Even today, though, many people are hard to read, and the variety of human responses to different situations and contexts makes it likely that we will all be at least sometimes misread. Unobserved or unobservable or incorrectly interpreted inputs are likely to limit predictions about human behavior forever.
The fact that Elizabeth bases her prediction of Darcy’s actions on an insufficient amount of information would fall under what the Princeton professors call “The 8 billion problem,” and her acceptance of information about Darcy from some people who have reason to dislike him and misrepresent him points to the broader issue of bias in datasets, which are themselves, as researcher Solon Barocas has pointed out, “artifacts of human intervention, not records imparted by nature itself.” In the context of AI predictive models, these issues, too, might lead to inaccurate predictions about particular people or groups.
Ironically, in Pride and Prejudice, Elizabeth herself initially fails to heed her own insight. Early in the book, when someone argues that country society offers limited opportunities for “studiers of character” because it is so “confined and unvarying,” Elizabeth answers, “But people themselves alter so much, that there is something new to be observed in them for ever.”
People change; learn; impact each other. Technologists might call that “drift.”
Of course, the proponents of AI/ML predictions claim specifically that vast datasets and carefully designed algorithms are an improvement over any Elizabeth’s analysis and will lead to predictions that are much more accurate than those made by human beings. As noted above, however, predictive models face many of the same limitations that human predictors do (in their essay, the Princeton professors list many more than the ones mentioned here). Unfortunately, in the case of algorithms, the limitations are often more hidden.
As Narayanan and Salganik stress, much of the current critique of predictive algorithms “has rarely contested the predictive analytics industry’s claim that machine learning methods are delivering great improvements in accuracy compared to human experts and traditional statistics. Questioning that assumption changes the debate completely.” (Note the reference to experts; part of the issue in the first part of Pride and Prejudice is that Elizabeth, though very smart and insightful, is very young and inexperienced, too. She, too, changes as she learns.)
We need to change, completely, the debate about the use of various AI tools to accurately predict individual human behavior. When it comes to anticipating the impact of predictive algorithms on society, write the Princeton professors, “[a] pitfall that’s just as common as failing to anticipate advances is to overreact by assuming that a breakthrough is just around the corner.” Another pitfall is to claim, hubristically, that it’s already occurred.
Photo: "Jane Austen's Desk w/ Quill @ Chawton" by akintsy_photo (cropped) is licensed under CC BY-NC 2.0